How to implement a Data innovation strategy for the healthcare sector
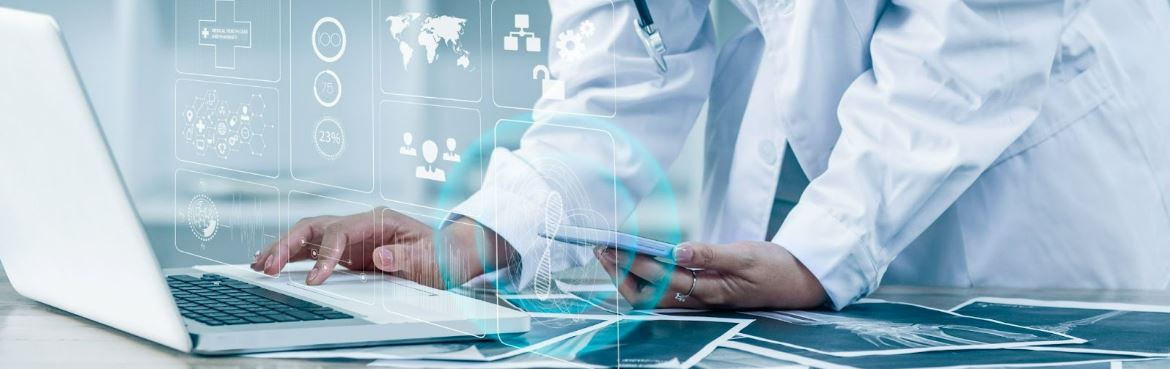
Reading Time: 3 minutes
The evolution of Data Science applicable to healthcare has been undergoing a major acceleration in recent years. Data has been applied robustly in this field for some time now, but with the development of increasingly advanced techniques in machine and deep learning and others more related to AI, such as natural language processing (NPL), the evolution of the value obtained from data is becoming exponential.
It is complicated to have all the necessary profiles in each phase of a project of this type since they have, by their very nature, a clear multidisciplinary component. In this sense, the support of Talent As a Service models to have the right talent at the right time is key.
Another fundamental element is to know which phases must be undertaken so that innovation in the healthcare data strategy delivers the necessary value in a safe way; all of them are important and necessary.
Define Clear Objectives and Goals
- Establish what you want to achieve with your analytics strategy: improve decision making, enhance customer experience, or develop new products.
- Align these objectives with the overall business strategy to ensure cohesion and relevance.
Build a Qualified Team
- Hire the Right Talent: Form a multidisciplinary team with the key profiles identified above: Data Scientists, Data Engineers, Data Analysts, NLP Specialists, Machine Learning Engineers, Explainable AI (XAI) Specialists, Federated Learning Experts and a Data Science Project Manager.
- Continuous Training: Invest in continuous training and development to keep the team updated with the latest trends and technologies (Analytics Insight) (MyGreatLearning).
- In this phase, having a talent partner (TaaS) that reduces the difficulties and risks to a minimum may be the best option if you do not have all the necessary talent in the organization. In addition, continuous training and qualification will be guaranteed.
Invest in the Right Tools and Technologies
- Data Infrastructure: Establish a robust data infrastructure using tools such as Hadoop, Spark and cloud storage solutions to handle large volumes of data efficiently.
- Advanced Analytics and Machine Learning Platforms: Use platforms such as Azure ML, TensorFlow, PyTorch and Scikit-learn to build and deploy machine learning models (Yale School of Medicine) (McKinsey & Company).
- Data Visualization Tools: Implement tools such as Tableau and Power BI for effective data visualization and reporting.
Develop a Data Governance Framework
- Establish policies and procedures for data management, including data quality, security and privacy.
- Implement compliance measures to adhere to relevant regulations and standards, such as the GDPR for data protection and the National Security Scheme (mandatory in the Spanish public administration).
- This phase is absolutely fundamental in a sector such as the pharmaceutical industry, where the high sensitivity of data is an element to be taken into account (SpringerLink).
Implement Agile Methodologies
- Use agile project management techniques to ensure flexibility and iterative progress. This allows for continuous improvement and rapid adaptation to changing requirements (McKinsey & Company)..
- Regularly review and adjust strategy based on performance metrics and feedback.
Leverage Advanced Analytics and AI
- Integrate machine learning and AI to gain deeper insights and automate decision-making processes. Focus on Explainable AI to ensure transparency and confidence in your models (Yale School of Medicine) (SpringerLink).
- Explore federated learning to improve privacy and security while effectively utilizing distributed data sources (McKinsey & Company). When developing a data strategy in an industry as heavily regulated as healthcare we must assess and manage any potential risks.
Foster a Data-Driven Culture
- Promote a data-driven mindset throughout the organization by fostering data literacy and making data accessible to all relevant stakeholders.
- Use storytelling with data to effectively communicate insights and promote informed decision making (Yale School of Medicine).
Monitor and Evaluate
- Continuously monitor the performance of the data strategy against defined objectives and KPIs.
- Use feedback loops to refine models and processes, ensuring continuous improvements and innovation (McKinsey & Company).
Scale and Innovate
- As the data strategy matures, explore new areas of innovation such as Edge Computing, real-time analytics and Data-as-a-Service (DaaS) models.
- Keep the company abreast of emerging trends and technologies to remain competitive and forward-looking (MyGreatLearning) (McKinsey & Company).
This sequence of phases will allow us to innovate and maximize the value of our data with an adequate risk control, obtaining key support in decision making.
References
10 Data Science Papers for Academic Research in 2024 .- Analytics Insight (2023)
Latest Trends in Data Science 2024 .- GreatLearning (2024)
What Does Natural Language Processing Mean for Biomedicine? .- Yale School of Medicine (2023)
Natural language processing in healthcare .- McKinsey & Company (2018)
Natural Language Processing for Health-Related Texts .- Sprinkler (2021)
Natural Language Processing in Health Care and Biomedicine .- Sprinkler (2013)
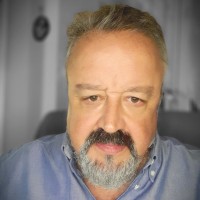